|
On April 8th, 2016, the North Carolina State University (NCSU) Women in Engineering (WIE) Student Chapter and the Elizabeth City State University (ECSU) IEEE-Geoscience & Remote Sensing Society (IEEE-GRSS) Student Chapter held a joint meeting on the campus of North Carolina State University. The program was part of the Distinguished Lecture Series and featured Dr. Melba Crawford, Associate Dean of Engineering for Research, Purdue University who's presentation was titled: "Hyperspectral Remotely Sensed Data: Opportunities and Challenges." (Event PDF Flyer) (Event Schedule)
Melba Crawford is a Professor of Engineering and Agriculture at Purdue University, where she is Director of the Laboratory for Applications of Remote Sensing and the Chair of Excellence in Earth Observation. She also serves as the Associate Dean for Research of the College of Engineering. Previously, she was a faculty member at the University of Texas at Austin, where she founded an interdisciplinary research and applications development program in space-based and airborne remote sensing. Dr. Crawford received the B.S. and M.S. degrees in Civil Engineering from the University of Illinois, Urbana, and the Ph.D. degree in Systems Engineering from Ohio State University, Columbus. |
|
ABSTRACT
Hyperspectral data provide new opportunities for exploiting chemistry specific features to characterize targets, perform atmospheric correction, cross calibrate sensors, and accomplish improved land cover mapping. Significant challenges remain due to correlated spectral bands, high dimensionality, and nonlinear spectral responses, as well as the quantity of data that is generated. Advanced methods in machine learning, including nonlinear feature extraction and active learning, are promising for analysis of hyperspectral data. Nonlinear manifold learning methods provide natural capability to both accommodate nonlinear scattering and practical, robust feature extraction methods in dynamic environments. Active learning techniques, that focus on developing informative training sets with minimal redundancy, promote greater exploitation of the information in both labeled and unlabeled data, while significantly reducing the cost of data collection. New developments for feature extraction via global and local manifold learning and strategies for active learning for classification and unmixing of hyperspectral data are presented, and the impact is demonstrated using testbed hyperspectral data from airborne platforms and the NASA Hyperion sensor. |
|
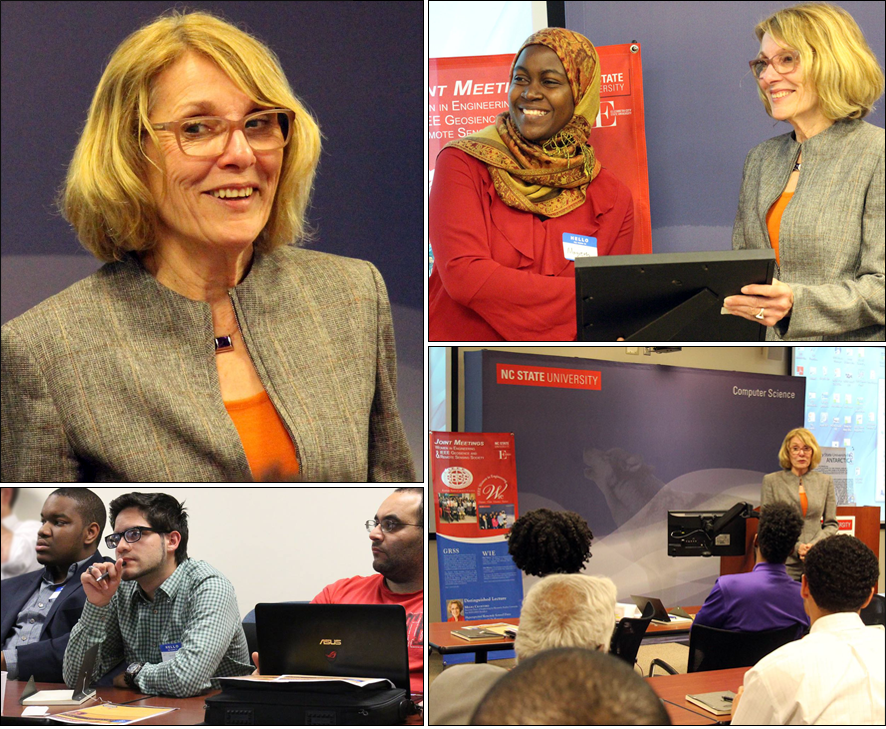
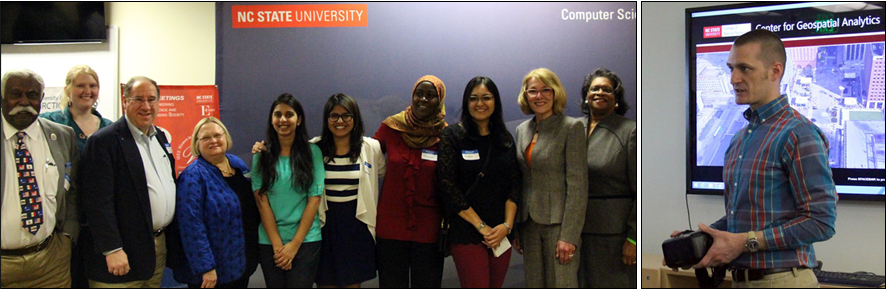
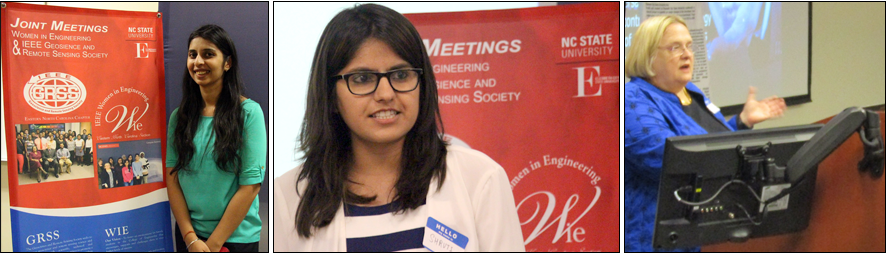
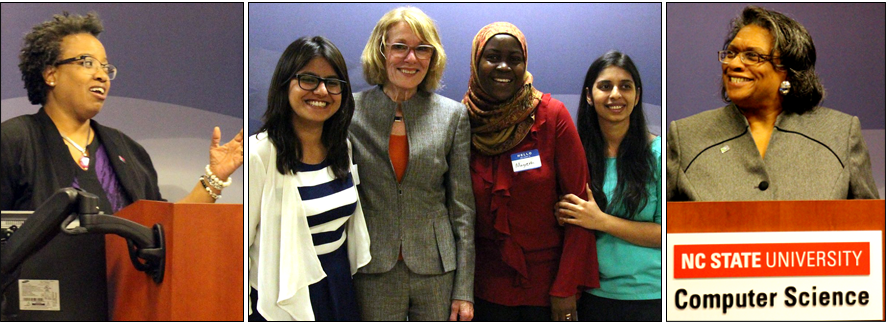
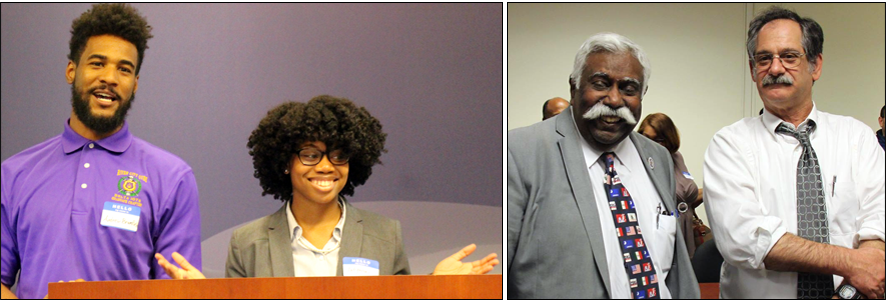 |
|
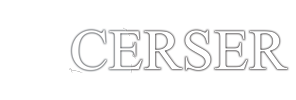 |
Center of Excellence in Remote Sensing Education and Research
1704 Weeksville Road, Box 672, Elizabeth City, North Carolina 27909
Phone (252) 335-3696 Fax (252) 335-3790
AaA REU grant number PLR-1263061 |
|
|
|